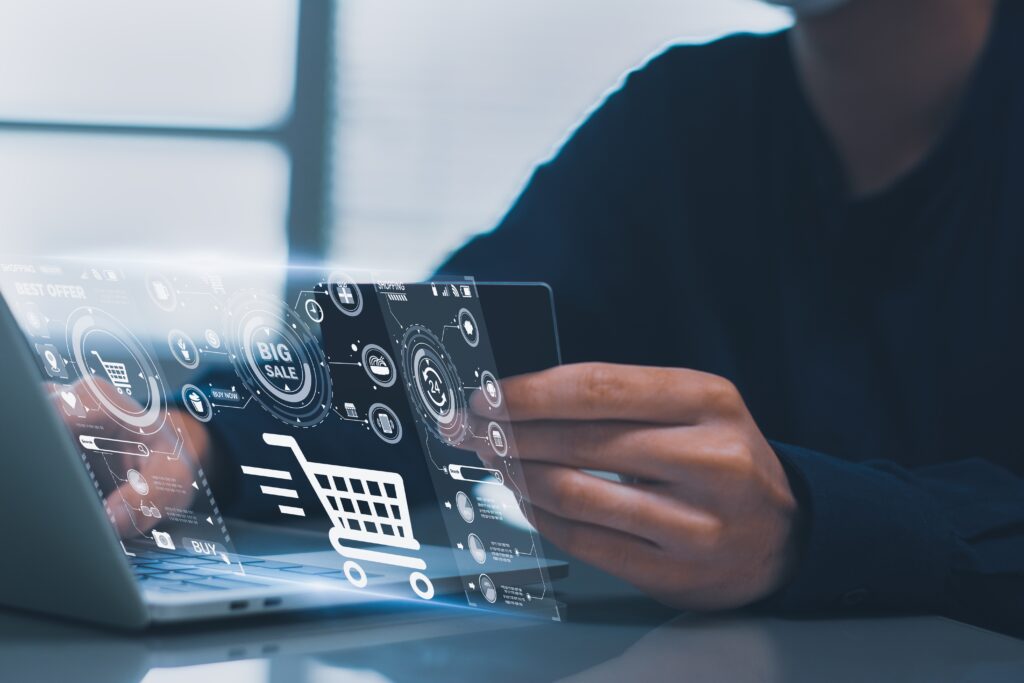
Exploring AI for F&B Benchmarking: What We Tried, What We Learned, and What’s Next
12 December 2024In collaboration with Improvability, ATNi explores AI for F&B benchmarking and its impact on scaling, efficiency, and more.
ATNi’s strategy refresh in 2023 heralded a renewed focus on thought leadership and innovation that leads to market transformation for nutrition. We wanted to rethink the way we produce, analyse, consume and publish our data. On everyone’s lips were two letters: A and I. Taking the lead in how AI can support benchmarking and drive a new dimension of value, ATNi began testing generative AI in late 2023.
The key question was: could generative AI help us conduct our benchmarking more efficiently, addressing challenges like accessing relevant public nutrition information or processing large amounts of text? More broadly, could AI for F&B benchmarking free up time for our research and communications teams to focus on impact rather than routine tasks? Could we look forward to assessing 100s of companies as opposed to dozens and reporting more frequently?
For a global non-profit like ATNi, investing in innovation is always a balancing act. How do you get a good return in the fastest moving technology space, with modest budget? Partnering with a startup was innovative in itself. This approach gave us the support we needed, both technical and visionary, allowing us to quickly build confidence and move to a pilot phase.
Our journey with Improvability began in late 2023. Improvability is a platform that uses generative AI to help businesses manage sustainability and supply chain processes. It addresses the growing demand for compliance with sustainability, supply chain and “know your customer” reporting requirements. By automating tasks such as report writing and data integration, it helps organisations navigate increasingly complex challenges, including regulations. The tool is designed to manage and query data securely, with features like personalised databases and detailed data extraction.
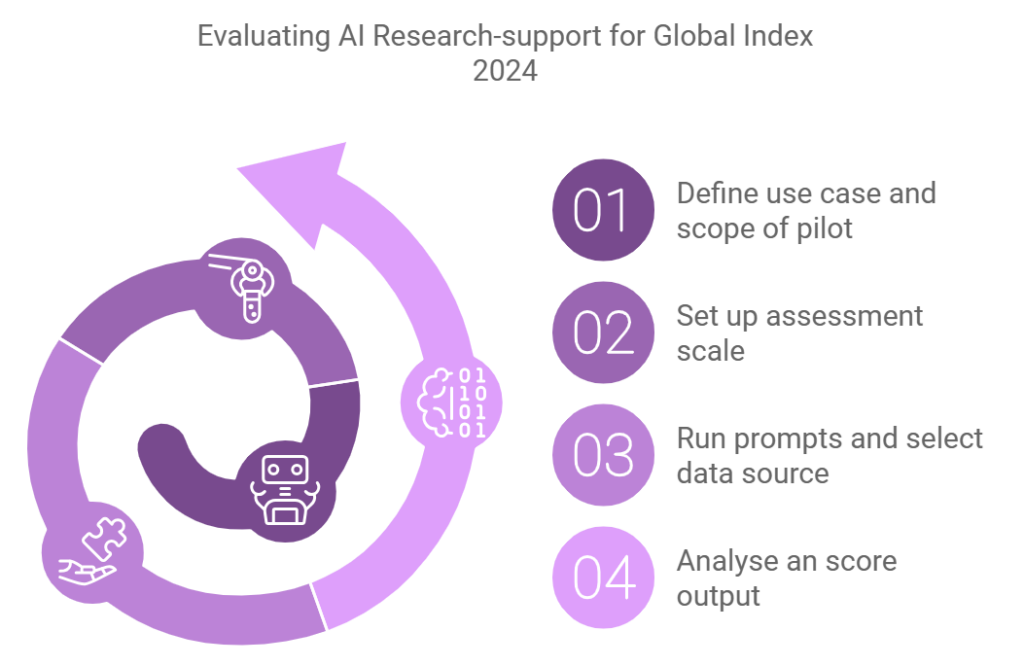
We started by analysing our existing research processes to identify the most relevant stages for automation and identified two use cases. The main one being around AI –assisted research applied to our Global Index research.
For this pilot, we relied solely on publicly available data, building on the data management solutions offered by the Improvability platform:
- Web search to query and retrieve information from the web efficiently
- Private Databases: to store historical ATNi methodologies, reports, and scorecards from past publications.
- Public database: A broad nutrition library based on international guidelines and key reference documents cited in ATNI’s Global Index methodology.
The process involved using indicators from ATNI’s methodology as prompts. The expected output included an answer option, a score based on the methodology, and a justification with citations and direct extracts of documents used as evidence.
Given time and resource constraints, we applied a simplified scale to evaluate the output, attributing scores ranging from 0 (Answer is completely incorrect or unreadable) to 3 (Answer correctly and comprehensively answers the question, not missing any major aspect).
Over the six months’ pilot, we saw an increasing rate of satisfactory output and obtained promising results. While the nature and time allocated to the pilot make it difficult to provide robust quantifiable outcomes, notably on time gained, we noted several key improvements in prompt structures that led to better results.
In what we called Prompt Generation 1, we followed an experimental approach. Analysts were not given strict guidelines to create the prompts. The Index methodology was stored in the Private database. It took an average of 1.5 prompts for the AI to reach a relevant answer. Outputs were on average given a score of 2 (Answer mostly answers the question but is missing or hallucinating a critical aspect). Internally, we concluded that it had reached an ‘intern’ stage but still needed a lot of guidance and verification.
In Prompt Generation 2, we adopted a more structured approach. The role of an ATNi researcher and the context of the research, especially the ATNi Global Index, were clearly defined. We systematically included the indicator questions, answer options, scoring guidance, and rationale based on the ATNi methodology. In addition, we fine-tuned our use of the different data sources. We kept building the Public and Private databases in parallel, increasing the nutritional knowledge of the solution.
Lastly, we elaborated on the task description, stressing the importance of justifying the selected answer options and citing specific extracts from evidence documents. This refinement in our process significantly improved the quality of our outputs.
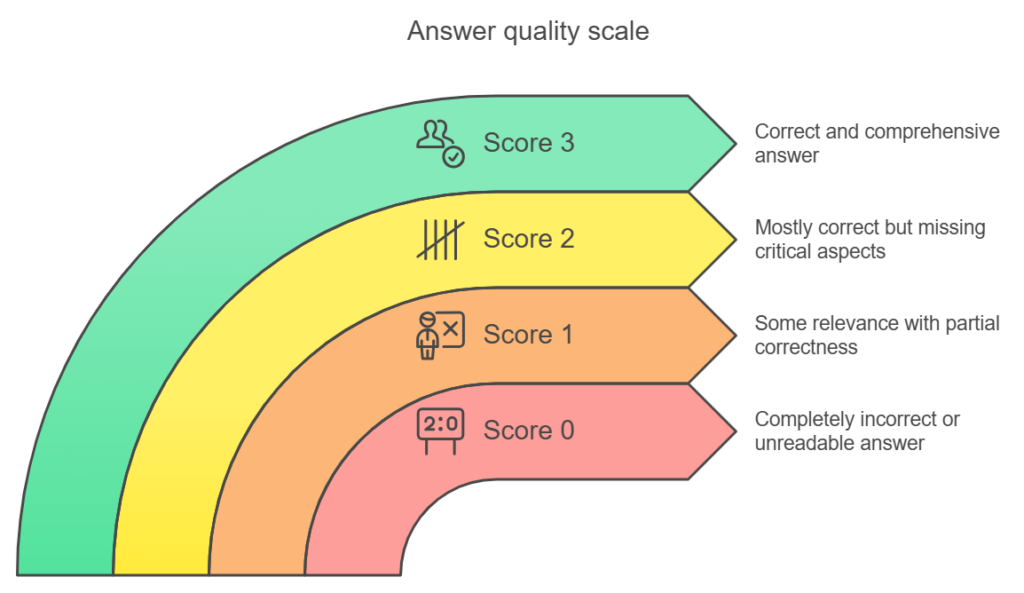
At ATNi, we are committed to responsibly applying generative AI for F&B benchmarking, ensuring it adds real value while keeping humans in the loop and in charge of quality. We aim to balance innovation with ethical considerations, continuously learning and adapting to emerging risks to drive positive change in the sector.
Empowering our research team with the Improvability platform will allow us to do more while liberating creative and thinking time for our team. From assessing more companies more frequently to going deeper into the issues our stakeholders find most valuable, and from presenting our findings in tailor made fashion to discovering new uses of the AI tool, how we work and how impactful we are is fast evolving.
Currently, ATNi is exploring, in partnership with Improvability, an AI-enabled Nutrition portal to provide consumers, regulators, and policymakers with insights into healthier, more sustainable food markets. With 57% of online content already partially processed by AI and projections reaching 90% by 2025, this initiative is timely.
A recent article by Dr. Ilia Shumailov from Oxford University, published in Nature, highlights the risk that AI models can fail when they continue to learn from data created by other AI systems. This underscores the growing importance of using quality data and information generated by humans to prevent bias in algorithms.
And this is exactly why ATNi has the ambition to keep safeguarding nutrition data and make it a reliable source for the public good.
To know more, watch this space …